Latest from Learning Center
Top Manufacturing Stories of 2024
Best Manufacturing Guides of 2024
What Is an Industrial PC?
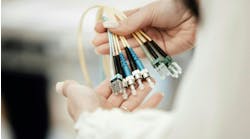
Do You Know Motors?
Introduction
Industrial IoT is at a transition point. While the number of connected devices continues to grow at a rapid rate, IoT is no longer just about monitoring equipment behavior. Increasingly, the value of IoT comes from collecting machine data and then analyzing and drawing out insights for quick action. Specifically, when IoT is combined with sophisticated analytics to rapidly process the vast amounts of data it receives, new use cases for industrial IoT emerge. This eBook discusses four use cases that you need to pursue for quick, actionable insights.
Download this article in pdf format.
Use Case 1: Remote Condition Monitoring and Remote Service
If the pandemic taught businesses anything, it’s the value of working remotely. During COVID-19 restrictions, onsite activities were significantly limited with travel bans and most facilities shut. That meant that routine visits to check the status of a machine or to service it was out of the question. Industrial IoT devices that can be accessed remotely offered several advantages during the pandemic. And even when things return to normal, businesses that make use of remotely connected IoT devices will continue to realize benefits. Let’s take a look.
Connected IoT devices make operational and performance data about equipment fully accessible and available remotely. That data can be used in several ways, including:
Monitoring equipment in real-time to spot potential problems:
When equipment operates outside of its normal temperature or pressure range, that may be a precursor to impending failure. Other metrics, such as custom-created key performance indicators (KPIs), can also be tracked. Many organizations are using such real-time status data to perform proactive maintenance rather than the more traditional calendar-based maintenance where equipment is inspected and serviced on a certain date. Being able to determine when a visit is needed can cut the costs associated with multiple routinely scheduled visits.
Performing some maintenance remotely:
A remote connection to equipment lets an OEM or service organization conduct some software-related maintenance without physically visiting the site. With the right connectivity and security features, companies can push software, firmware, and service pact updates using such a conduit. Additionally, equipment often can be remotely restarted, which frequently is all that is needed to reset an improperly performing unit. This remote maintenance reduces the travel costs that would be billed if these processes had to be done onsite.
Leveraging predictive capabilities:
Enhanced benefits can be realized when AI or ML-based analytics are applied to real-time and historical data from IoT devices. The sophisticated analysis can be used to predict problems by spotting trends that might lead to failure. By making inferences about the failure of an asset, an OEM or service organization can identify parts or equipment that are likely to fail before their scheduled replacement and maintenance time. With the ability to do this, spare parts do not need to be overstocked. They can be ordered as needed. Additionally, such insight can be used to trigger action before a problem occurs. While any site visit includes travel costs, airfare for unscheduled trips is much more expensive than when a company has the luxury of planning ahead.
New business models:
OEMs can leverage the benefits of using connected IoT devices and analytics to develop new business models. Rather than just selling equipment, they can offer premium service contracts based on remote monitoring and management capabilities.
Knowing that proactive maintenance can extend the life of an installed unit and spot problems before they happen, preventing downtime, an OEM can offer more stringent performance and uptime guarantees. Such guarantees quantified in service level agreements (SLAs) can be offered at a premium price.
An additional opportunity is to offer an “as a service” model for equipment where customers of installed equipment are charged by its use. Equipment ranging from an MRI system to an industrial welder can be billed by their output. The often-cited example of this model that defined the field early on was RollsRoyce’s Power-by-the-Hour service that offered a complete engine and accessory replacement service on a fixed-cost-per-flying-hour basis.
Download this article in pdf format.
Use Case 2: Performance Monitoring and the Connected Shop Floor
IoT data and its analysis can provide full transparency of how machines are operating, allowing proactive corrective actions to be taken to maintain the highest productivity and to maintain machine health. Insights from IoT data can be used for operational purposes to plan maintenance and spot problems in the making. The data also can be used more strategically to optimize operational efficiency.
Without IoT, gaining knowledge about a machine’s status and performance required a physical visit to collect basic operational information. IoT can provide detailed data about machine performance in real time.
This information can be used in numerous ways to optimize the performance capability and availability of machines. Alarms can be triggered when a system operates outside of its normal state. For example, if a machine’s temperature or pressure exceeds operating specifications, action can be taken to address the issue, such as throttling down the machine to bring it back into normal operating conditions. IoT data also can be used for better and more dynamic planning of service activities.
The Connected Shop Floor
Analysis of the data can help optimize the performance of machines, whether for a single machine or a complete production line. One use would be to calculate individual KPIs based on the data to optimize important figures, such as overall equipment efficiency, quality, and output. Such information allows an organization to compare the performance of different machines and plants.
An example is to put performance data, quality rate, speed of machines, and availability (up, down for maintenance, etc.) together into overall equipment effectiveness (OEE) or overall measure of the productivity of an entire line.
The IoT data and the related performance indicators can provide a wealth of information. Such data can then be analyzed to look for patterns and understand the inter-dependencies impacting OEE and production. If a low-performing machine is identified, its operating parameters could be adjusted to bring it back up to specifications. Or it might be an indication that a critical part is degrading and must be replaced.
IoT performance monitoring data can be used in other ways. Integrating it into other processes and workflows can deliver great value.
It could be as simple as tying an IoT performance alert into a service organization’s trouble ticket system. Or an application could marry the IoT performance insights to an ERP system to order a replacement for a failing part.
Use Case 3: Closed-Loop Digital Twin
Digital twins are virtual copies of physical assets. They use simulations, artificial intelligence, and machine learning on ingested data from IoT sensors to provide valuable insights into the operation of a device. Closed-loop digital twins use, in near-real-time, live performance data pushed from IoT devices. That data is fed into the digital twin of the product and the digital twin of production.
Closed-loop digital twins are increasingly being used in industrial settings to give OEMs new ways to design, monitor, and manage products by allowing simulations to become as precise as possible. Additionally, digital twins can provide organizations that service or operate equipment data a way to understand how equipment is performing in its setting and to troubleshoot problems.
Applications of digital twin technology serve different purposes. For industrial operations, a closed-loop digital twin may be used to monitor and improve operations, evaluate what-if scenarios, and help improve the design of a device. These application areas include:
Operational twin:
In many cases, equipment problems are due to incorrect usage. For example, a device might be operating out of its normal temperature or pressure range, or it might be running at a higher speed than recommended. If unaddressed, the issue can impact the device itself, perhaps leading to an unexpected breakdown or aggressive deterioration. Using a digital twin of the part, data analysis of a device’s current state might help identify such out-of-specification operation conditions. Simulations could help explore whether pushing a device’s performance or running it in its current environment is okay. If not, changes could be made to optimize the device’s current performance in a working environment.
Predictive twin:
A closed-loop digital twin can be used to do what-if analysis. For example, a manufacturer might look at trade-offs: If I increase the operating speed of a device, what is the immediate improvement in output? Then, use the same simulation to figure out the impact of that speed increase on the equipment. Would it need more frequent service? Would it fail faster? A cost analysis could then be done comparing the net revenue increase in a production boost by running the device at a higher rate versus the increased cost of maintaining the equipment or replacing it more frequently.
Loopback to product design and marketing:
A product design team might use data collected about the equipment used in the field to improve or tweak the product or add new features based on its current use. Does it perform better than expected? Perhaps, a more economically designed version would suffice. Similarly, it may turn out that a device in certain environments outperforms stated specifications. Marketing and sales might share that information with other customers in the same environments.
Additionally, a complex system or industrial process can be represented by a digital twin that integrates models of the parts that comprise the system. For example, a complex assembly line might include a digital twin of robotics systems that place items on a conveyor belt, another for a mill, yet another for a welding tool, and more. A composite digital twin with these elements would let a manufacturer get deeper insights into the end-to-end processes. Adjustments could be made in the virtual model to see what impact the changes would have on the finished product, production rates, quality, and more.
Use Case 4: Energy Management (for Production)
Industrial applications require lots of energy. In the U.S., the industrial sector accounted for more than a third of the total U.S. energy consumption in 2019. More and more, organizations are looking to industrial IoT for help in better understanding energy use, how to manage it, and how to reduce consumption and costs.
The ability to access energy information and effectively analyze real-time data to extract key indicators is a crucial factor for successful energy management. The problem most industrial organizations face is that they can only monitor aggregated energy consumption for an entire facility. They lack real-time visibility into energy efficiency on the shop floors.
That’s where industrial IoT comes in. A suitable IoT implementation can provide granular data about consumption needed to monitor usage. Analysis of that data can detect abnormal energy consumption patterns and quantify energy efficiency gaps.
A plant might use IoT to monitor the energy performance for each machine on a shop floor, capturing data such as power consumption and process operating parameters like temperature, pressure, operating speed, etc. Data collected from monitoring is used to understand the level of energy use at that moment.
That data can be examined for usage patterns upon which actions are taken. A plant may find that an older piece of equipment is using much more energy than a new model. A simple calculation might find that it makes sense to replace the unit with a more energy-efficient model.
Another use of the data is to time-shift heavy energy use. Monitoring via IoT may detect machines that have high consumption during peak energy times. In locations that have time-of-day or variable pricing rates based on demand, such information can be used to run those machines at times when costs are lower.
There are other uses for IoT and energy management besides helping to reduce energy costs. A facility can gather and analyze energy usage data and use the information to develop corporate sustainability goals. Similarly, the data and insights can be used to forecast biweekly energy requirements and share that information with a power provider. And manufacturers can prove to the government they have applied technology and practices to optimize energy consumption to get tax deductions.
IoT Implementation Choices for Any Use Case
For any technology solution, the first choice a company must make is whether to build or buy. That is certainly the case with industrial IoT.
The advantage when building any solution is that an organization gets to define and implement every aspect to their own liking. For simple systems, that may be a good option. But building an IoT solution is a complex task.
The first thing to consider is that a solution has hardware, software, and connectivity elements. A company would need expertise in each area to determine needs, evaluate various solutions, and trial their use. And then, additional work is needed to integrate each of the elements and optimize end-to-end performance.
Many organizations find they lack internal expertise across all areas. If that is the case, a company will need to hire new employees and or plug the gaps with contractors. It takes time for groups to form, get trained, and begin working together as a team. The result can be a long time to value.
Because a solution includes multiple elements, an organization undertaking a new effort may not be able to appreciate the scope of the technical challenges and dependencies of an IoT project. This can, at best, lead to missed deadlines for any project’s timeline, and at worst, result in a solution that does not meet the organization’s requirements. Similarly, a lack of expertise often leads to budget problems for technical projects. There can be unknown costs to update software or address new security risks as they emerge.
Perhaps the biggest negative on the build side is that there are constant technological advances. Building a solution today is not the end of the story. In many cases, solutions will need to scale over time.
Additionally, new hardware, software, and connectivity developments are happening all the time. Today’s solution may need to quickly be updated when an IoT device, analytics software, or communications service comes out. Organizations may not have the skills, budget, or available staff time to accomplish this.
Countering these building negatives are the buying benefits. An IoT solution is not a simple case of selecting and managing a single technology. Today’s “buy” option is an investment, a tightly integrated solution where the elements, which are dependent on one another, optimally work together. A suitable IoT solution might also include data management, analytics services, and security.
The value of a “buy” approach is that the organization is freed from the complexities of using IoT and can focus on its own core business functions, which enables it to deliver value faster.
An additional benefit of a “buy” approachꟷsuch as using MindSphere, Siemens' industrial IoT as a service solutionꟷis that an organization can quickly and easily connect the IIoT solution to machinery on the factory floor. And it future-proofs any IIoT initiative to accommodate perpetual innovation and growing complexity.